Over the past couple years, the investment world has been filled with talk about generative AI’s potential to revolutionize all industries and make workers more productive. However, as generative-AI applications have been deployed on a larger scale, numerous questions have begun to bubble up regarding the timing of the benefits and actual monetization of the technology. Given the hundreds of billions of dollars in AI-related capital expenditures (capex) deployed by mega-cap technology companies in recent years, investors are increasingly starting to scrutinize the path to an adequate return on investment in the form of more meaningful revenue contributions from generative-AI applications.
In our view, the benefit to software companies may not be materially realized until at least 2025 (if not 2026), largely a result of how revenue is recognized from a subscription business model. Further, if companies do not realize the productivity and efficiency gains from the increased expenses related to premium AI-capable software platforms, management teams may pull back on the spending and revert to legacy subscriptions without AI capabilities as they seek to optimize expenses.
That said, we believe the overall trend of building out the infrastructure needed to support widespread AI acceptance will likely continue. More specifically, the data-center footprint that supports AI applications will likely expand, and we believe big technology companies will continue allocating capital toward purchasing hardware (that is, servers; semiconductors, to include graphics processing units (GPUs) and central processing units (CPUs); networking equipment; and heavy equipment) as well as land (to densify and expand their data-center footprint).
Finally, as new versions of large-language models have scaled by doubling the amount of parameters and compute capacity, the energy requirements to support these sophisticated models have increased dramatically. Accordingly, a key area of strategic focus that has emerged among large hyperscale providers has been securing reliable sources of environmentally friendly power. In this report, we also discuss the liquid-cooling technologies that data-center operators are increasingly pursuing in order to reduce energy consumption levels.
Power supply is key to operating a data center, so most facilities have redundancies in place to help avoid downtime. The cause of outages within data centers usually center around a few categories, including design; capacity issues; hardware failures (due to overheating or cooling issues); human error; environmental events; or even power disruptions.
Risks posed by power outages
Given the amount of data points and information collected as more and more devices are connected to the cloud, businesses have become dependent on data centers. Revenue may therefore be lost as a result of the outage, or data-center operators may even be required to reimburse customers for lost revenue while the outage occurred. Further, the data-center operator may be exposed to reputational risk in the event of an outage — the industry may view the company as an unreliable option going forward as an outage could potentially indicate a lack of adequate controls and measures in place to provide reliable services or to even ensure the security the information within the data center. Other potential types of risks or losses that could result from extended downtime include business interruption and lower productivity. According to a 2024 study from the Uptime Institute, one in ten data center outages was categorized as serious or severe. While eliminating outages altogether may be impossible, the study showed that data-center outage risk could be mitigated by having proper policies and procedures in place.
The uses of data centers continue to evolve, especially with the development and widespread acceptance of generative-AI applications. The number of parameters used in developing these applications has exploded over the past few years. Parameters are variables used in large-language models during the training phase to optimize the results and performance. These parameters are what the model uses to learn and adapt and to provide an outcome to a query. It is believed that more parameters equates to better answers, and thus more powerful models. While more parameters will likely translate into a better model, it certainly equates to more costly training and potentially higher expenses to cool, and thus run, efficiently.
Just a few years ago, models utilized millions of parameters when training their models. For example, the parameter count for Alphabet’s Bidirectional Encoder Representations from Transformers (BERT) was 110 million in 2018.1 That figure has exploded, with the third version of OpenAI’s ChatGPT (ChatGPT-3) utilizing more than 175 billion parameters during its training phase.2 Although OpenAI has not disclosed the number of parameters utilized in the fourth version (ChatGPT-4), we believe the pool of parameters is likely multiples larger than prior versions. This hypergrowth of variable usage has resulted in a much more complex process to provide an outcome to an AI-related query. It has also contributed to GPUs becoming one of the most important components used for training generative-AI-based large language models.
Liquid-cooling technology’s role in addressing challenges
Given the aforementioned surge in compute-intensive data processing (which is related to the large number of data points used to process a single AI-related query), two significant challenges have arisen within the data center environment — energy consumption and heat dissipation. Proximity to a reliable power source is necessary to keep the data center up and running efficiently. Even more important is the dissipation of heat exuded by the servers and other hardware. Various techniques are used to cool the equipment, and previously, the most common technique was air cooling. However, technologies have advanced, and liquid cooling has become more efficient than air cooling for dissipating the heat and keeping the servers running efficiently. Most data centers are expected to transition from air cooling to liquid cooling over the next few years.
As discussed above, data-center outages can be caused by multiple environmental factors — one of these is excess heat produced by high-density hardware and servers. In our view, liquid-cooling technology can help prevent data center outages. Some of the benefits of deploying liquid-cooling technology for data centers include reducing overall operating expenses, improving power-usage effectiveness, and reducing carbon emissions from data centers powered by fossil fuel.
The primary drivers of elevated electricity demand in data centers are computing and cooling requirements — computing represents 40% of electricity demand while traditional air cooling (that is, HVAC and computer room air-conditioning units) represents about 40% of energy consumption. The remaining 20% is associated with the power-supply system and other information-technology-related equipment. According to Hewlett Packard Enterprise Co.’s estimates, however, liquid-cooling technology in data centers can help reduce overall energy consumption by 30%.
Energy usage in data centers 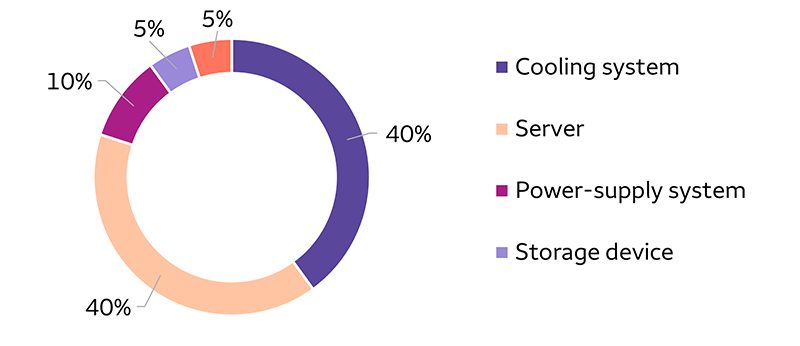
Source: ABB Group. Data as of May 2022.
Liquid cooling uses a liquid coolant to absorb and remove heat generated by data centers’ hardware components. The two main categories of liquid-cooling technology consist of direct-to-chip liquid cooling and immersive liquid cooling. Direct-to-chip liquid cooling involves applying the liquid coolant over the hotter chip components, and with this method, fans are still required to generate enough air cooling to remove excess heat. Meanwhile, the immersive liquid-cooling method entails submerging entire servers vertically in a dielectric fluid that cools the server components directly. This method has the advantage of eliminating the need of fans for air cooling.
1 Jacob Devlin, Ming-Wei Chang, Kenton Lee, and Kristina N. Toutanova; "BERT: Pre-training of Deep Bidirectional Transformers for Language Understanding," 2018.
2 Language models are few-shot learners, OpenAI, May 28, 2020.
Often, the amount of power that a hyperscale data center requires to operate is equivalent to that of a moderately sized city. As previously noted, access to reliable power is a necessity for data centers to operate effectively and efficiently, especially as applications become more complex with added AI capabilities. We believe technology companies will need to develop and implement cutting-edge methods of cooling the servers, including liquid cooling, to increase energy efficiency as the use of complex applications grows through the use of AI. Liquid-cooling technologies appear to be addressing some of the largest challenges within the evolving data-center environment, dissipating the heat generated from an increasing number of racks, servers, and other hardware. We therefore believe that companies with deep experience in these technologies will be well positioned as data centers’ information-technology infrastructure continues to be built out.
Generally speaking, data centers are owned and operated by colocation companies or large technology firms, including cloud vendors. While colocation companies typically lease out the data centers and provide network capacity to their customer base, large cloud vendors tend to utilize the infrastructure for their own purposes. Although hyperscalers have been customers of colocation companies in the past, we continue to see more building out their own network of data centers for their own usage.
We believe there will continue to be significant data-center capacity brought online over the next few years, driven by the rapid growth of AI applications and sustainable data-center demand. Advancing technologies and end-user applications (such as gaming, streaming, and increased usage of large-language models) should continue the trend of rising data-center demand. According to Synergy Research Group, hyperscale data-center capacity is expected to triple by the end of 2029, driven by high-compute AI-related applications — that expansion could take the form of new data centers or existing data centers that are expanded or retrofitted to increase their capacity.
Cloud vendors need to perform a great deal of work to manage the infrastructure of the cloud. In order to provide a reliable network, these companies need to invest heavily in the hardware (servers), virtualization technology, and overall network infrastructure — that is, the backbone — of the cloud. The cloud vendors are tasked with managing and maintaining the overall cloud infrastructure as well as making their services available to their cloud customers. As the chart below shows, the pace of spending from the top cloud providers is expected to increase over the coming years as they continue to build out infrastructure in support of AI.
Capex spending by largest hyperscalers 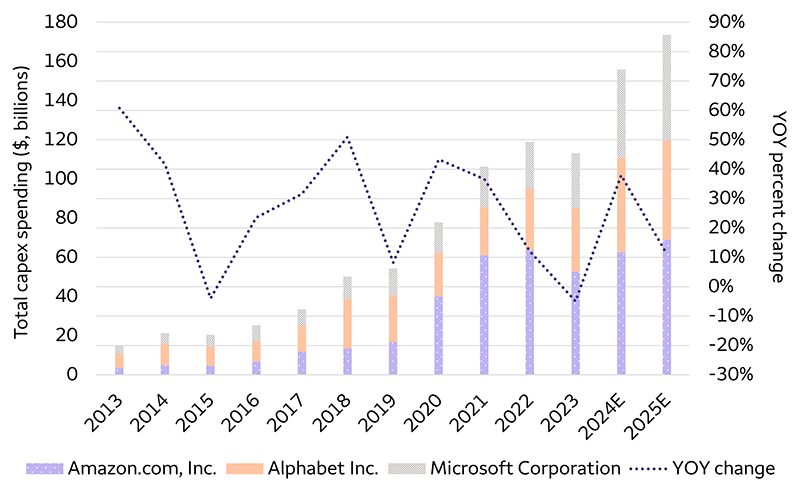
Sources: FactSet and company filings. Data as of July 15, 2024. YOY = year over year. E = estimated.
Past performance is no guarantee of future results.
Although enterprises continue to migrate workloads to the cloud, we believe the ongoing secular shift to the cloud remains one of the most powerful themes within software and represents a generational shift. In our view, we are likely in the early years of a multi-decade transition as businesses realize the efficiency and economic benefits to moving large amounts of data from on-premise to the cloud.
Impacts on the Information Technology sector
We continue to see long-term investment opportunities for companies supporting the highly compute-intensive nature of AI-focused data centers. Modern advances in GPU architecture such as parallel processing have been crucial in powering modern AI servers. GPU chips are used to train the majority of deep neural networks and large-language models used to perform deep-learning AI tasks, including generative AI. We believe leading GPU chip suppliers are well positioned to benefit from the proliferation of AI and high-density rack servers in modern data centers. Also, we expect leading semiconductor companies that sell networking and storage chips to benefit from supporting the networking silicon needs of data centers.
More generally speaking, we see potential benefits for PC and smartphone demand related to the development of generative AI. As we emerged from the pandemic and consumers shifted their preferences from consumption of goods to services and experiences, PC unit demand declined 17% in 2022 and fell once again by 14% exiting 2023. The PC original equipment manufacturers (OEMs) are, however, optimistic about AI PCs — that is, PCs with AI integrated into both the hardware and software — as a potential catalyst to drive an overall PC replacement cycle sometime during the second half of 2024 and into 2025. Gartner estimates 54.5 million AI PCs will ship in 2024, despite expectations of worldwide PC units to show signs of stabilization in 2024, with forecasts for approximately 3% year-over-year unit growth. This year, we also expect more premium smartphones to be capable of running on-device generative-AI models. Gartner estimates that generative-AI smartphones will reach 240 million units exiting 2024. For 2024, Gartner estimates worldwide smartphone shipments will stabilize, growing 4% YOY to 1.2 billion units.